1. Data peeking
Data peeking, or the practice of looking at test results before the test has concluded, can lead to biased results and false positives in A/B testing.
Since Bayesian A/B testing enables continuous monitoring philippines girls telegram and updating of the test results as new data is collected, you’ll need to avoid looking at the results too early to reduce the chances of drawing false conclusions from prematurely ending the test.
To avoid data peeking issues in Bayesian A/B testing, follow these steps:
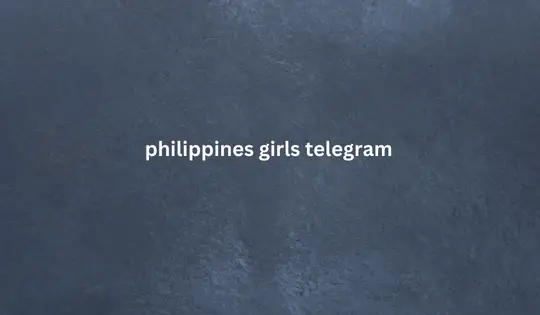
Resist the temptation to examine the data until you achieve the required sample size per variation.
This approach secures accurate and reliable Bayesian A/B test results, reducing the likelihood of false positives.
2. Prior sensitivity
Prior sensitivity refers to the effect of different prior assumptions on the test results in Bayesian A/B testing.
Performing a sensitivity analysis by modifying the prior and observing result changes can mitigate the influence of a particular prior assumption on the test’s conclusions.
This method helps evaluate the conclusion’s robustness and the impact of the prior on the A/B test’s final outcomes.
When selecting priors for Bayesian A/B testing, it’s important to take into account prior beliefs or knowledge about the parameters of interest. A non-informative prior, such as a uniform or Jeffreys prior, which assigns equal probability to all possible values, is a popular option.